We have a dataset of 40 data of concrete, density and strength.
The focus here is Exploratory Data Analysis (EDA) of the dataset:
statistics of the 2 features
histograms, scatter plots
coefficient of correlation and linear regression between the data
At first, we import the main libraries that we will use
IMPORT MODULES
1 import numpy as np 2 from OpenAIUQ import Stat as sta
In Line 1 we import numpy, in Line 2 we import the module Stat from OpenAIUQ. The prefix of Stat is sta.
LOAD THE DATASET (40 data of concrete data)
1 dataset=np.loadtxt('concrete40.txt') 2 features_all=['density','strength'] 3 concrete=sta.data2(dataset,features=features_all) 4 concrete.disp_summary()
In Line 1 we load the dataset (40 data of concrete density and concrete strength), like a 2darray (40,2). In Line 2 we name the features (list). In Line 3 we associate the object concrete (type=sta.data2) to the dataset. To check the main statistical properties of the sample in Line 5 we use the method disp.summary.

It is seen that the concrete density ranges from min=2411 kg/m3 to max=2488 Kg/m3. This implies that a good range of analysis for the density can be x=[2400, 2500] Kg/m3. The sample mean is m=2444.93 Kg/m3, with coefficient of variation v=1% showing a low degree of variability. The concrete strength ranges from min=49.90 MPa to max=69.50 MPa. This implies that a good range of analysis for the density can be y=[50, 70] MPa. The sample mean is m=60.14 MPa, with coefficient of variation v=8%, which is a typical value of uncertainty for strengths.
The attribute concrete.d of sta.data2 is a list of objects sta.data1. They are stored in the same order of the columns of the dataset. Each one of them collects all the information of the single features.
1 density=concrete.d[0] 2 edges=np.arange(2410,2500,10) 3 density.plot_hist(bins=edges) 4 density.ax.set_xticks(edges) 5 density.ax.set_xlabel('density $(kg/m^3)$'); 6 fig_density_hist=density.fig
In Line 1 we associate to the object density the first element (data type=sta.data1) of the list concrete.d. In Lines 2-6 we plot the corresponding histogram.

1 strength=concrete.d[1] 2 edges=np.arange(50,72.5,2.5) 3 strength.plot_hist(bins=edges) 4 strength.ax.set_xticks(edges) 5 strength.ax.set_xticks(edges) 6 strength.ax.set_xlabel('strength $(kg/m^3)$'); 7 fig_strength_hist=strength.fig
In Lines 1-7 we plot the histograms of the feature strength (data type=sta.data1)

Once we have described the univariate data (density and strength) we need to describe the correlations between the features.
SCATTER PLOT
1 concrete.plot(x0=0, y0=1)
In Line 1 we apply the method plot to the object concrete (sta.data2). The parameters x0=0 and y0=1 mean that we choose to represent in the x-axis the first column (index=0) and in the y-axis the second column (index=1). If no other option is selected, the method plot provides the scatter plot.

CORRELATION
1 concrete.plot_corr(heatmap='yes',output='yes')
In Line 1 we apply the method plot_corr to the object concrete (sta.data2). The option heatmap='yes' prints the heatmap of the correlation matrix, while the option output='yes' prints the matrix of correlation on the screen.

SCATTER PLOT AND REGRESSION
1 concrete.plot(x0=0,y0=1,regression='yes')
In Line 1 we apply the method plot to the object concrete (sta.data2). The option regression=yes is included. Therefore the regression line is represented.

These figures describe the presence of correlation between concrete density and strength. The value is r=0.44 (mid value of correlation). It is positive, this means that the strength increases with the density, and this makes sense from physical point of view.
SCATTER PLOT, HISTOGRAM AND AND REGRESSION
1 concrete.plot(x0=0,y0=1,hist='yes',regression='yes')
In Line 1 we apply the method plot to the object concrete (sta.data2). The options hist=yes and regression=yes are included. In this case the plot provides scatter plot, histograms of the chosen features, and represents the regression line. This plot is based on jointplot of seaborn.

PAIR PLOT
1 concrete.plot(pair='yes')
In Line 1 we apply the method plot to the object concrete (sta.data2). The option pair=yes show the pairplot of seaborn, where scatter plots and histograms are paired in the plot.
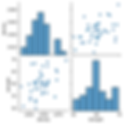